A (sort of) optimistic case for AI
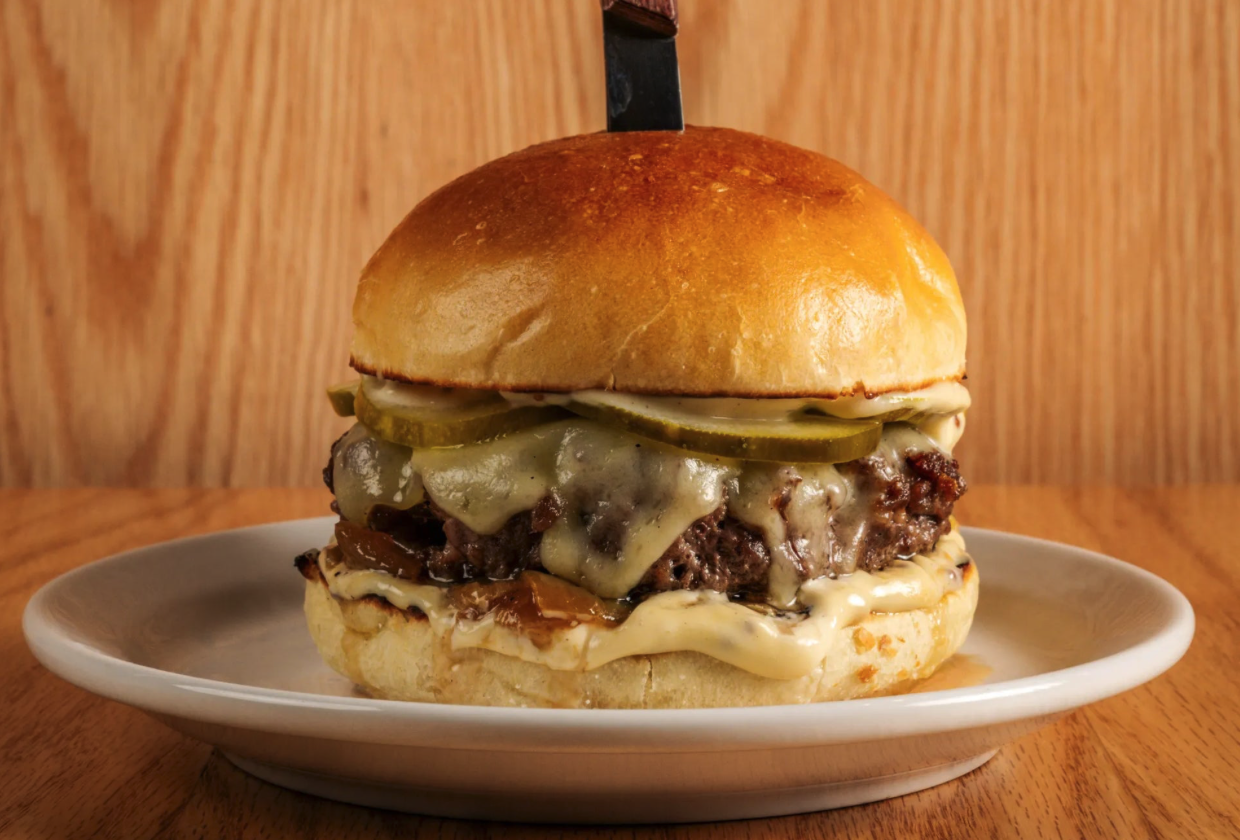
I read Tressie McMillan Cottom’s recent piece for the NYT with interest. In it she argues that AI is part of a string of “mid” technology. It’s a useful piece because it isn’t just about the technology itself, but the discursive ends to which the ideas of particular technolgies are put:
A.I. is already promising that we won’t need institutions or expertise. It does not just speed up the process of writing a peer review of research; it also removes the requirement that one has read or understood the research it is reviewing. A.I.’s ultimate goal, according to boosters like Cuban, is to upskill workers — make them more productive — while delegitimizing degrees. Another way to put that is that A.I. wants workers who make decisions based on expertise without an institution that creates and certifies that expertise. ~Expertise without experts~.
I am very ambivalent about AI. I seem to vacillate wildly between thinking it’s all overblown hype and that it’s actually a really big deal.
Last year I spent months working on a cover story for The Walrus in which I tried to gently puncture the hype around AI. My focus was mostly on the concept of intelligence, in large part because what started to bother me about the AI hype was that intelligence was often positioned as a quantity rather than a quality. The problems of the world are like mounds of data that just require ever greater intelligence to solve and, say, homelessness will just get sorted once we have enough compute.
But even in the relatively brief time since that story came out, my perspective on AI has changed somewhat.
A key thing about AI discourse is to make a distinction between the technology and its externalities. It is fun to have a machine make you a limerick; it is bad, as the joke goes, to boil the oceans to do so. Like many things — driving a car, or moving to the suburbs, or voting for a politician who will cut one’s taxes — the immediate experience in one’s own life of that choice is vastly different from the aggregate effect of millions making the same choice together.
But - here’s where I am cautiously, gingerly optimistic about AI: in my experience, what we’re currently calling “AI” can change how one approaches the idea of data and its potential for manipulation.
A small, very stupid example. Local magazine Toronto Life frequently produces listicles — here is where to eat in this neighbourhood and so on. I read one, and then found myself wishing that they put all those places on a map. Then I remembered ChatGPT. I plugged the article text into ChatGPT and asked it to pull out the names and addresses of the listed places. I then plugged that list into Excel, got it to separate out the information into columns, created a .CSV file, and then plugged that file into Google Maps. Voila: there was my map. (I later learned that I could just get ChatGPT to automate that penultimate step).
Yeah, sure, I could have done something similar in a slightly more manual way in 2015, too. But prior to what we’re calling “AI”, I would have never even thought to do that. That is what I am surprised by. I am interested in tech, but not terribly technical. But the capacity to have a machine “do things with data” changed how I approached information in general; it is now a thing that even I can extract*, manipulate, reform, repurpose.
Many people “on my side of the aisle” are often vociferously, adamantly anti-AI. I understand the reasons why. Yet I can’t help but feel a sort of optimism about the capacity of the tech to help me do things I would have never thought to before. It’s not that I couldn’t do those things before, mind you. It’s just that I wouldn’t. And that is often a key difference. Just as I would have never published my own zine — but did write my own blog once that possibility arose due to the Web — AI drops barriers to doing certain things. Only this time it isn’t publishing, but futzing with information.
McMillan Cottom is clear that the kind of change I am describing in how one thinks of data doesn’t simply emerge out of AI itself. You don’t become skilled or proficient in something because of the tech. Rather, you have to know what you need to know:
Mark Cuban… imagined an A.I.-enabled world where a worker with “zero education” uses A.I. and a skilled worker doesn’t. The worker who gets on the A.I. train learns to ask the right questions and the numbskull of a skilled worker does not. The former will often be, in Cuban’s analysis, the more productive employee.
The problem is that asking the right questions requires the opposite of having zero education. You can’t just learn how to craft a prompt for an A.I. chatbot without first having the experience, exposure and, yes, education to know what the heck you are doing. The reality — and the science — is clear that learning is a messy, nonlinear human development process that resists efficiency. A.I. cannot replace it.
That is true! You have to know what to ask.
And yet, the other day, I asked ChatGPT to create an Excel formula for a financial thing I was pondering, and it worked brilliantly. Again, I could have learned this in theory — could have found tutorials or taken a “for Dummies” book out of the library — but I never would have thought to even try because it felt too far out of my ken. It was the ease, rather than the absolute possibility, that initiated my experimentation.
I don’t quite know what this means for AI, or anything else. The history of technologies that make things more convenient on an individual level but have other, deleterious aggregate effects (again: cars) is one in which most people choose convenience, consequences be damned. If there is a way to combat that, I haven’t discovered it yet.
But all the same, there’s at least something in that feeling of “oh maybe I can do this thing too” which is interesting and promising to me. Perhaps that also makes me part of the problem. That’s a thing I am still figuring out.
*yes yes, I know, talking about the extractive as a good thing in the 2020s sounds funny. I hope what I’m saying here is clear